Philip Stark
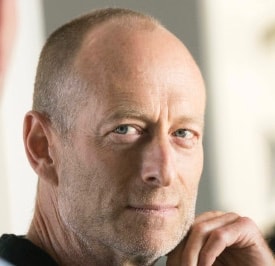
Specialty areas
Data Reduction and Inference (inverse) Problems, Primarily in Physical Science.
Biography
My research centers on inference (inverse) problems, especially confidence procedures tailored for specific goals. Applications include the Big Bang, causal inference, the U.S. Census, climate modeling, earthquake prediction and seismic hazard analysis, election auditing, endangered species, epidemiology, evaluating and improving teaching and educational technology, food web models, health effects of sodium, the geomagnetic field, geriatric hearing loss, information retrieval, Internet content filters, nonparametrics (constrained confidence sets for functions and probability densities, permutation methods), reproducibility, resilient and sustainable food systems, risk assessment, the seismic structure of Sun and Earth, spectroscopy, spectrum estimation, and uncertainty quantification for computational models of complex systems. Methods I developed for auditing elections have been incorporated into laws in California, Colorado, and Rhode Island. Methods for data reduction and spectrum estimation I developed or co-developed are part of the Øersted geomagnetic satellite data pipeline and the Global Oscillations Network Group (GONG) helioseismic telescope network data pipeline. Numerical optimization is important to my work; I’ve published some software. I’m also interested in nutrition, food equity, and sustainability. I am studying whether urban foraging could contribute meaningfully to nutrition, especially in “food deserts,” starting by investigating the occupancy, nutritional value, and possible toxicity of wild foods in the East Bay; see the Berkeley Open Source Food Project.
Consulting and expert witness topics have included Truth in advertising, behavioral targeting, the U.S. Census, clinical trials, construction defects, consumer class actions, credit risk models, election contests, environmental litigation, equal protection, First Amendment protections, geochemistry, intellectual property and patents, jury selection, trade secrets, employment discrimination, food safety, import restrictions, insurance and reinsurance litigation, Internet content filters, mortgage-backed securities, natural resource legislation, oil exploration, pharmaceuticals and nutraceuticals, product liability class actions, public utilities, quality control, Qui Tam (whistleblower) cases, risk assessment, sampling in litigation, signal processing, toxic torts, wage and hour class actions, warranties, water treatment, and white-collar crime
SticiGui is an online introductory Statistics “text” that includes interactive data analysis and demonstrations, machine-graded online assignments and exams (a different version for every student), and a text with dynamic examples and exercises, applets illustrating key concepts, and an extensive glossary. In 2007, SticiGui became the basis of the first online course (in any subject) taught at UC Berkeley. With Ani Adhikari, I co-taught a series of introductory statistics MOOCs in spring 2013. Nearly 53,000 students enrolled in the first course, of whom more than 10,600 finished and nearly 8,200 received a certificate of completion.
Philip’s Stark CV